
What is important for CIOs considering adopting GenAI?
김채욱
While entering
“GenAI,” which has recently taken the world by storm, is receiving high attention at the forefront of technological innovation. GenAI is expected to change the world with its potential and influence. Amidst this wave of change, corporate chief information officers (CIOs) in particular may be deeply concerned about whether to adopt GenAI and how to do it.
From the CIO's point of view, adopting GenAI is not just a technical choice; it can be said that it is a strategic decision that will influence the future of the company. It is impossible to avoid thinking about whether to adopt GenAI, how to approach it, and what specific benefits companies can obtain through it.
In this article, we will check why it is difficult to adopt GenAI and discuss how companies can make good use of GenAI.
LLM? FM? GenAI?

First, I will explain the categories and concepts of GenAI. GenAI exists in various forms and functions, and in order to understand it, it is important to clarify the relationship between LLM (Large Language Models), FM (Foundation Models), and GenAI.
LLM refers to large-scale language models and is an artificial intelligence model mainly trained based on text data. These models have excellent performance in generating sentences and understanding contexts by maximizing natural language processing power through vast amounts of data. For example, models such as GPT-3 are representative examples of LLM.
The larger concept that includes this LLM is FM, or Foundation Models. FM refers to a basic model that can be used for various artificial intelligence tasks by learning not only text but also various types of data such as images and sounds. FM is a multi-purpose artificial intelligence model that has a wider range of application possibilities than models specific to specific tasks.
Finally, GenAI, which is the biggest concept, encompasses a variety of generative artificial intelligence technologies, including FM. GenAI refers to artificial intelligence that can perform not only text generation, but also various creative tasks such as image generation, music composition, and code writing. In other words, GenAI is a comprehensive artificial intelligence concept that includes both LLM and FM functions, and is capable of more than that.
In this way, LLM, FM, and GenAI are structured so that each concept forms a dependency relationship and gradually expands. By understanding these divisions and dependencies, companies can more accurately select and utilize the artificial intelligence technology they need.
GenAI is still difficult to implement
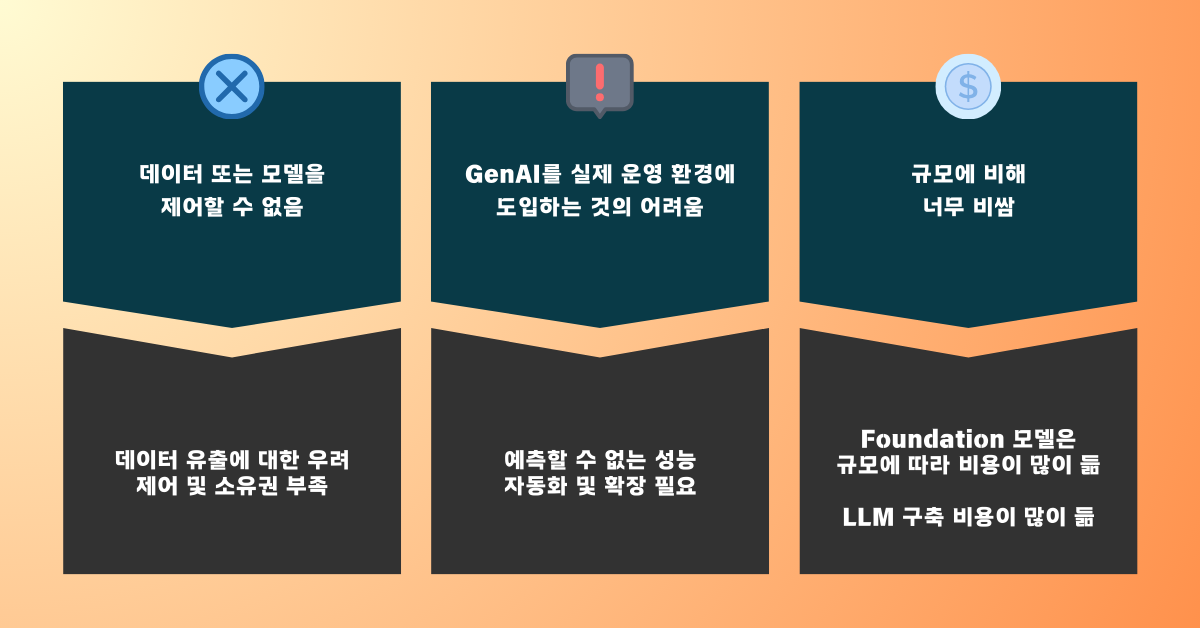
Adopting GenAI comes with a number of challenges. First, the lack of control over data or models raises concerns about data breaches and lack of control and ownership. GenAI systems require vast amounts of data, but they need a clear solution on how to securely manage and protect this data. Since data breaches are directly related to a company's credibility, this can be a major obstacle.
Second, introducing GenAI into a real operating environment is very difficult. Along with unpredictable performance issues, there is a high demand for automation and scalability. GenAI models can perform well during development and testing, but performance degradation can occur due to various variables in actual operating environments. Addressing this requires advanced technical capabilities and continuous monitoring and tuning.
Third, GenAI adoption is too expensive for its scale. In particular, Foundation Models (FM) cost a lot of money depending on the scale, and Large Language Models (LLM) are also expensive to build. This can be a huge burden, especially for small businesses, and finding cost-effective alternatives is essential.
Essential elements for adopting GenAI

Adopting GenAI requires more than just a good model. First, full ownership and control over models and data is essential. This addresses data breaches and security issues, and enables companies to fully manage data and models.
Second, the quality of a consistent operating environment that enables faster and more stable deployment across multiple use cases is critical. GenAI models need to work reliably in a variety of environments, and this requires a high level of technical support and management.
Finally, you need a reasonable cost structure that allows you to cost-effectively build a large-scale LLM. This is an important factor, especially for small and medium-sized enterprises, and it is necessary to prepare a plan to build and operate a high-performance GenAI system at an appropriate cost.
Let's take a closer look at these three elements.
1. Complete control: Maintain full ownership, control, and security of models and data
An important aspect of adopting GenAI is maintaining full ownership, control, and security of models and data. This is an essential element for companies to effectively utilize GenAI and minimize risks such as data breaches.
First, you need to be able to securely store, fine-tune, and pre-train models with your own data. Businesses must be able to securely manage sensitive data internally without relying on the outside, and be able to fine-tune or pre-train models if necessary. This can reduce the risk of data breaches and maintain a higher level of security.
Second, ownership of both data and models is important. By owning and controlling data, companies can clearly set the scope of use of the data and modify or update it if necessary. Also, by owning ownership of the model, companies can control the direction and pace of development of AI solutions themselves.
Third, unified rights and governance from data to models must be possible. This helps maintain data and model consistency and control the flow of data at every step. Integrated rights and governance enable companies to manage data quality, use data in accordance with policies, and comply with regulatory requirements.
2. Operating environment quality: Enhanced LLMOps for faster and more reliable deployment
One of the key points in adopting GenAI is the improved LLMOps operating environment quality for faster and more stable deployment. This allows GenAI's performance and efficiency to be maximized.
First, it is necessary to ensure accurate and safe model results. Data quality control and continuous model verification are important so that GenAI models can produce accurate predictions and results. This enables companies to build reliable AI solutions.
Second, it must have built-in monitoring capabilities. The ability to monitor the performance and status of models in real time is central to GenAI operations. This allows you to quickly detect and respond to unexpected model errors or performance degradation.
Third, you must be able to manage the entire LLM cycle on a single platform. Operational efficiency can be greatly improved by managing all processes such as data collection, model building, deployment, and relearning on a single platform. This integrated management environment ensures consistent data flow and efficient workflows.
3. Affordable: Fast model training at a lower cost
The ability to quickly train models at a low cost is key to strengthening a company's competitiveness.
First, you need the ability to build your own LLM with an optimized stack through prior learning. Businesses can build an LLM more efficiently by leveraging pre-trained models and combining them with optimized infrastructure. This reduces initial investment costs and enables rapid adoption of AI solutions.
Second, the ability to train multi-billion parameter models in just a few days is critical. By utilizing the latest technology, vast amounts of data can be processed and learned quickly, which can dramatically reduce model building time. This helps companies respond quickly to changing markets.
Third, it must be possible to reduce training costs. With an efficient model training process and optimized infrastructure, existing high-cost training processes can be drastically reduced. This can greatly reduce the economic burden of adopting AI.
Finally, it is important to provide an optimized LLM to reduce deployment costs. To minimize costs during deployment, you must be able to operate efficiently using an optimized model. This enables sustainable AI operations and results in long-term cost savings.
Data management, an essential element for making good use of GenAI

In order to make good use of GenAI, it is essential to manage and accumulate high-quality data and build a data lake house that can be easily accessed. Data Lakehouse provides an open and integrated architecture that can be used for analysis and AI model training by integrating diverse data.
First, the data lakehouse is based on an open data lake that can store all source data in an integrated manner. Various types of data such as logs, text, audio, video, and images can be stored and managed efficiently. This makes it possible to manage various data sources on a single integrated platform.
Additionally, the data lakehouse provides integrated data storage for reliability and sharing. Solutions such as Delta Lake create an environment where data can be stored reliably and easily shared as needed. This plays an important role in maintaining data consistency and fostering collaboration among diverse teams.
Unity catalogs are used for integrated security, governance, and catalog management. This enhances data security and governance, and enables systematic data management. You can maintain security by setting detailed data access rights and tracking data usage history. This systematic data management is essential to ensure data reliability and ensure regulatory compliance.
Delta live tables are used for ETL and real-time analysis. It enables real-time data processing and analysis, and can derive insights based on the latest data. This enables companies to make quick and accurate decisions.
Workflows are provided for orchestration. It automates data pipelines and enables efficient data processing. This streamlines complex data processing processes, making it possible to utilize data more quickly.
Finally, it includes DataBrix SQL for data warehouses. It's a powerful tool for efficiently querying and analyzing structured data. You can quickly process large amounts of data and gain meaningful insights.
According to MIT Technology Review Insights, DataBrix, which pioneered the data lakehouse architecture in 2020, is an important solution adopted by 74% of global companies today. This enables companies to efficiently manage vast amounts of data and build an environment optimized for GenAI model learning. For the successful introduction and use of GenAI, it is very important to secure high-quality data management and accessibility through a data lake house.
DataBrix's data intelligence platform

The combination of Data Lakehouse and GenAI goes beyond a simple synergy and brings innovation to the way companies utilize data and apply artificial intelligence. Data Lakehouse provides an open and integrated architecture for all data, which enables efficient management and storage of various source data (logs, text, audio, video, images, etc.). A data lakehouse enables integrated data storage for stability and sharing, real-time analytics and ETL, and integrated security and governance.
When GenAI is added to this, the situation evolves even further. GenAI supports easy expansion and use of data and artificial intelligence. Beyond simply storing and managing data, GenAI helps create real insights and innovative solutions using data.
What is created by combining these two elements Data intelligence platformThis is it. Data intelligence platforms enable the democratization of data and AI across the organization. This means going beyond simple data management and analysis, utilizing data in real time, deriving immediate insights through AI, and supporting all departments and teams within the organization to make data-driven decisions.
Effectively adopt GenAI through DataBrix's data intelligence platform!

유용한 SaaS 관리 콘텐츠가
업데이트될 때마다 알림을 받아보세요.
PoPs는 아래 내용에 따라 귀하의 정보를 수집 및 활용합니다. 다음의 내용을 숙지하시고 동의하는 경우 체크 박스에 표시해 주세요.
1. 개인정보 수집자 : 메가존클라우드㈜
2. 수집 받는 개인 정보
[필수]이메일
3. 수집/이용 목적
- PoPs 뉴스레터 제공
4. 보유 및 이용 기간 : 개인정보 수집일로부터 3년(단, 고객 동의 철회 시 지체없이 파기)
※ 개인정보 이용 철회 방법
- 안내 문자 등의 동의 철회를 이용하는 방법 : 이메일 수신 거부 링크 클릭 또는 안내 문자 내 수신거부 연락처를 통한 수신 거부 의사 통보
- 개인정보 처리 상담 부서
- 부서명: Offering GTM Team
- 연락처:offering_gtm@mz.co.kr
※ 동의거부권 및 불이익
귀하는 동의를 거절할 수 있는 권리를 보유하며, 동의를 거절하는 경우 상기 이용 목적에 명시된 서비스가 제공되지 아니합니다.
[선택] 개인정보 수집·이용 동의 안내 (마케팅 활용 및 광고성 수신 정보 동의)
PoPs는 아래 내용에 따라 귀하의 정보를 수집 및 활용합니다. 다음의 내용을 숙지하시고 동의하는 경우 체크 박스에 표시해 주세요
1. 개인정보 수집자 : 메가존클라우드㈜
2. 수집 받는 개인 정보
[필수]이메일
3. 수집/이용 목적
- PoPs 뉴스레터 제공
※ 본 동의문에는 이메일을 활용한 광고성 수신 동의 내용이 포함되어 있습니다.
4. 보유 및 이용 기간 : 동의 철회 시 까지
※ 개인정보 이용 철회 방법
- 안내 문자 등의 동의 철회를 이용하는 방법 : 이메일 수신 거부 링크 클릭 통한 수신 거부 의사 통보
- 개인정보 처리 상담 부서
- 부서명: Offering GTM
- 연락처: offering_gtm@mz.co.kr
※ 동의거부권 및 불이익
귀하는 동의를 거절할 수 있는 권리를 보유하며, 동의를 거절하는 경우 상기 이용 목적에 명시된 서비스가 제공되지 아니합니다.